The Machine Learning Process: A Comprehensive Guide for Business Success
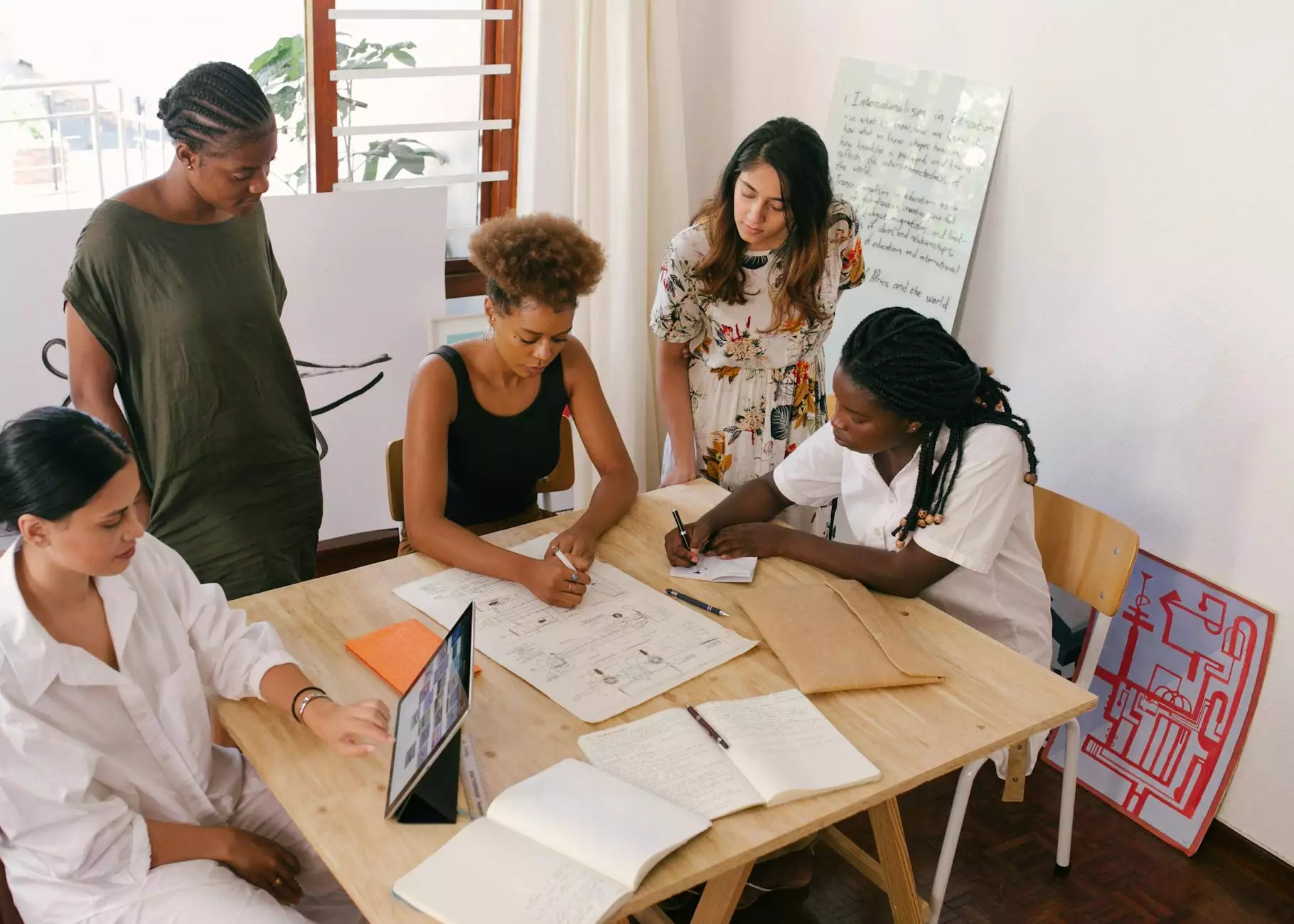
In the rapidly evolving realm of technology, machine learning has emerged as a groundbreaking innovation with the potential to revolutionize businesses across various industries. This powerful tool enables machines to learn from data, identify patterns, and make decisions with minimal human intervention. Understanding the machine learning process is essential for businesses looking to harness the full potential of this cutting-edge technology.
Introduction to Machine Learning
Machine learning is a subset of artificial intelligence that focuses on developing algorithms and models that allow computers to learn from and make predictions or decisions based on data. These algorithms are designed to improve their performance over time without being explicitly programmed.
The Machine Learning Process
The machine learning process typically consists of several key stages, each essential for building robust and accurate models:
- Data Collection: The first step in the machine learning process involves gathering relevant data from various sources. High-quality data is crucial for training accurate models.
- Data Preprocessing: Once the data is collected, it needs to be cleaned, transformed, and prepared for analysis. This step ensures that the data is consistent and free from errors.
- Feature Engineering: Feature engineering involves selecting, extracting, and transforming the most relevant features from the dataset to enhance the model's performance.
- Model Selection: Choosing the right algorithm or model is critical for the success of a machine learning project. Different models are suited for specific types of data and tasks.
- Model Training: During this stage, the selected model is trained using the preprocessed data. The goal is to adjust the model's parameters to minimize errors and improve accuracy.
- Evaluation: The trained model is evaluated using testing data to assess its performance. Metrics such as accuracy, precision, and recall are commonly used to evaluate model performance.
- Deployment: Once the model is deemed satisfactory, it is deployed into production where it can make predictions or decisions based on new incoming data.
Applications of Machine Learning in Business
Machine learning has extensive applications in business operations, marketing, finance, healthcare, and more. Some common use cases include:
- Forecasting: Machine learning models can be used to forecast sales, demand, and other business metrics, helping organizations make data-driven decisions.
- Customer Segmentation: By analyzing customer data, businesses can segment their customers and personalize marketing strategies to improve customer engagement.
- Anomaly Detection: Machine learning algorithms can detect unusual patterns or outliers in data, helping businesses identify potential fraud or anomalies in real-time.
- Recommendation Systems: E-commerce platforms use machine learning to provide personalized product recommendations based on customer preferences and behavior.
Conclusion
As businesses strive to stay competitive in a data-driven world, understanding the machine learning process and leveraging its capabilities can provide a significant edge. By implementing machine learning models effectively, businesses can improve decision-making, optimize processes, and enhance customer experiences.
Explore the endless possibilities that machine learning offers, and unlock the potential for growth and innovation in your business today!
explain about machine learning process